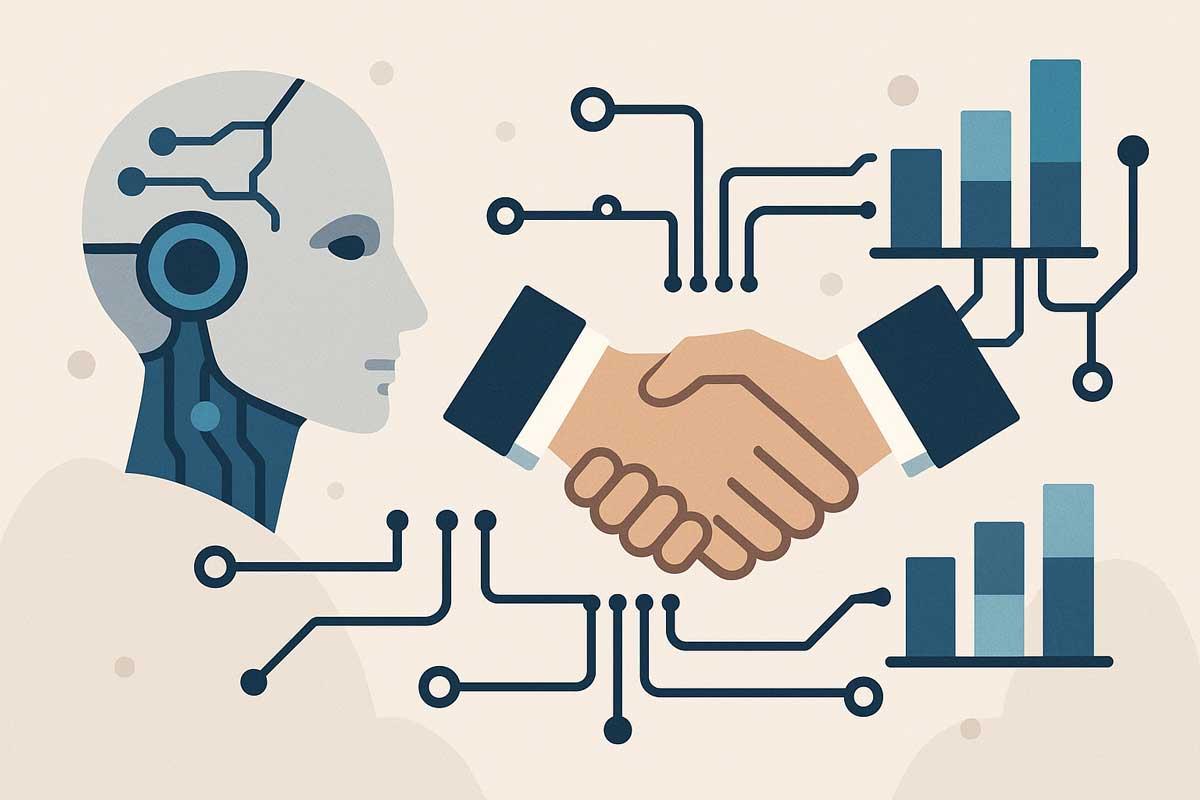
Role Of AI In Mergers and Acquisitions
The landscape of mergers and acquisitions is undergoing a seismic shift, driven by the rapid advancement of artificial intelligence (AI) technologies. As organizations increasingly harness the power of AI and particularly generative AI (GenAI), the process of identifying, valuing, and executing M&A deals is set to evolve dramatically. This article explores the implications of AI on M&A, the emerging trends, and how businesses can leverage these technologies to enhance deal-making processes.
1. Understanding Generative AI and Its Role in M&A
1.1 What is Generative AI?
Generative AI refers to algorithms that can create new content, ranging from text and images to data models, by learning from existing data. GPT-3 and similar technologies exemplify this capability, potentially transforming how businesses communicate, analyze, and make decisions.
1.2 How Generative AI Influences M&A
Generative AI tools can assist in various aspects of M&A, including:
- Data Analysis: Processing vast datasets to identify potential acquisition targets or assess the health of a company.
- Due Diligence: Streamlining the due diligence process by automating document review and risk assessment.
- Valuation: Enhancing financial modeling and forecasting, providing more accurate valuations of potential targets.
2. Enhancing Deal Sourcing with AI
2.1 Predictive Analytics
AI can leverage predictive analytics to identify emerging trends and potential acquisition targets. By analyzing industry trends, competitor performance, and market signals, companies can uncover hidden opportunities.
Chart 1: Projected Outcomes
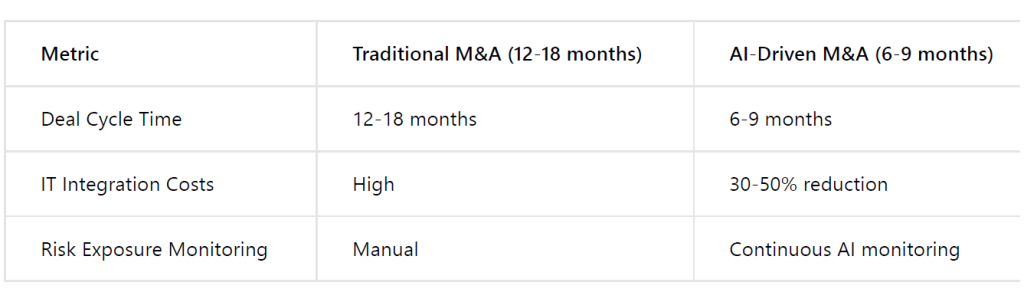
2.2 Market Sentiment Analysis
AI-powered tools can analyze market sentiment using social media, news articles, and financial reports to gauge the reputation and performance of potential targets. This holistic view can provide invaluable insights for decision-makers.
3. Transforming Due Diligence with Automation
3.1 Document Review and Analysis
Generative AI can enhance the due diligence process by automating document analysis, flagging potential risks, and summarizing critical information.
3.2 Risk Assessment
AI models can evaluate the risks associated with a target acquisition by analyzing historical data, market conditions, and regulatory factors. This helps investors make informed decisions.
Table 1: Traditional vs. AI-Driven Due Diligence Processes
ProcessTraditional MethodAI-Driven MethodDuration4-8 weeks1-2 weeksHuman Resource RequirementHigh (multiple teams)Low (automated via AI tools)AccuracySubject to human errorData-driven insights
4. Enhanced Valuation Models
4.1 Data-Driven Valuations
AI allows for the creation of sophisticated financial models by using diverse datasets, improving accuracy in revenue projections and valuation metrics.
4.2 Scenario Analysis
Companies can simulate various market scenarios using generative AI to understand potential outcomes and make better-informed decisions about valuations.
5. Integration and Post-Merger Management
5.1 Cultural Assessment
AI tools can assess cultural compatibility between merging organizations by evaluating employee sentiment and survey data, helping ensure smoother transitions post-merger.
5.2 Performance Monitoring
Post-merger, AI can continuously analyze performance metrics, providing real-time insights into integration success and guiding adjustments as needed.
Chart 2: AI in Post-Merger Integration Monitoring
MetricTraditional MethodAI-Driven MethodFrequency of ReportingMonthlyReal-timeDepartments InvolvedMultiple, decentralizedCentralized AI dashboardInsights GeneratedLimitedComprehensive, actionable
6. Challenges and Ethical Considerations (Continued)
6.1 Data Privacy and Security
The use of AI in M&A processes raises concerns about data privacy and compliance with regulations such as GDPR and CCPA. Organizations must ensure that the data used in AI models is collected, stored, and processed in compliance with legal frameworks to safeguard sensitive information.
6.2 Bias in AI Algorithms
AI models can sometimes reflect biases present in the training data. Companies must be vigilant in evaluating the algorithms to ensure fair and unbiased decision-making throughout the M&A process.
6.3 Human Oversight
While AI can enhance efficiency, human oversight remains critical. Decisions should not be made solely based on AI recommendations; human expertise is necessary to interpret AI outputs and factor in qualitative aspects that may not be captured by data.
7. The Future Landscape of M&A in an AI-Driven World
7.1 Democratization of AI
As AI technologies become more accessible, smaller firms with limited resources can leverage AI tools to compete more effectively in the M&A landscape. This democratization could lead to increased competition and innovation across various industries.
7.2 Continuous Learning and Adaptation
AI models can evolve through continuous learning, adapting to market changes and emerging trends. This allows organizations to stay ahead of competitors and respond effectively to shifting landscapes.
7.3 Collaborative Ecosystems
The future will likely see an increase in collaborations between technology firms and traditional companies. By combining expertise in AI and industry knowledge, organizations can create more effective M&A strategies.
8. Conclusion
As the M&A landscape continues to evolve, the integration of AI technologies will fundamentally reshape how deals are sourced, assessed, and executed. Leveraging generative AI can unlock new efficiencies, enhance analytical capabilities, and provide insights that were previously unattainable.
For organizations willing to embrace these technologies, the potential for strategic advantage is immense. However, navigating the accompanying challenges—such as data privacy, algorithmic bias, and the need for human oversight—will be paramount to ensuring successful outcomes.
In this AI-driven world, companies that leverage digital intelligence while maintaining ethical standards and human insight will likely thrive in the competitive landscape of mergers and acquisitions.
Leave a Reply